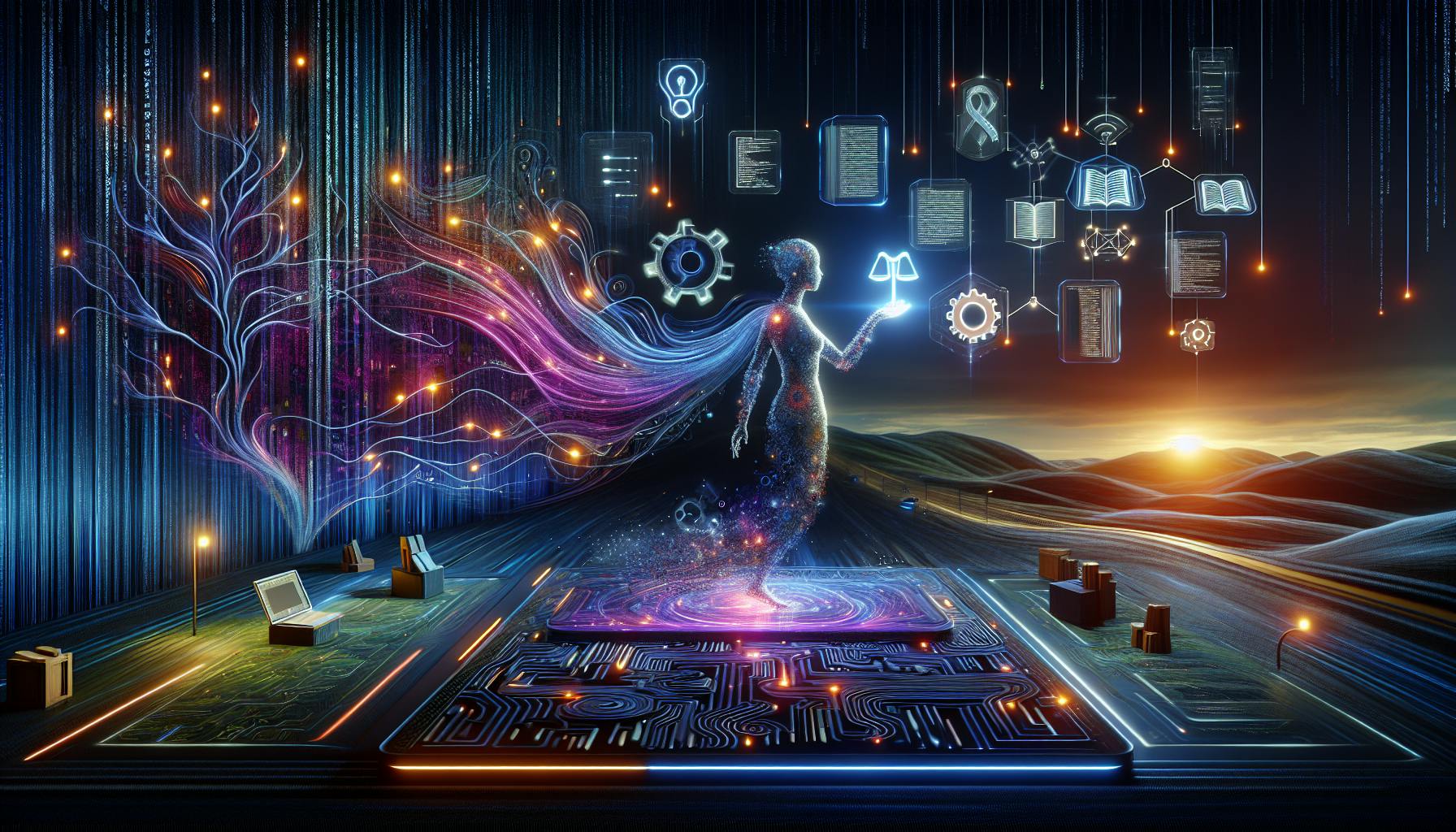
Discover SIMA, the new generalist AI agent by Google DeepMind. Learn about its language understanding, versatility, ethical approach, challenges, and future work.
Google DeepMind has unveiled a groundbreaking AI agent named SIMA, standing for Scalable Instructable Multiworld Agent. This agent represents a significant advancement in AI, capable of navigating and learning in diverse virtual environments through observation and instruction. Here's a quick rundown of what makes SIMA so unique:
- Generalist AI: Unlike previous AIs focused on specific tasks or games, SIMA is designed to adapt and learn across various 3D game worlds.
- Language and Image Understanding: It combines image-language models with video prediction to understand and interact with its environment effectively.
- Learning Methods: SIMA employs a blend of reinforcement learning, imitation learning, and self-supervised pretraining to enhance its capabilities.
- Versatile Applications: While initially trained in video game environments, the potential applications of SIMA extend to real-world tasks.
- Ethical Approach: DeepMind emphasizes ethical training, ensuring SIMA's applications promote helpful and respectful interactions.
This AI's ability to learn and adapt without game-specific programming highlights a move towards creating more versatile and general-purpose artificial intelligences.
Understanding SIMA
SIMA is a smart tool from Google DeepMind that can figure out what to do in many different 3D games by looking at the screen and listening to instructions. It's built with two main parts that help it understand and act in these game worlds:
Core Components
- Image-language model: This part helps SIMA understand pictures and relate them to words. It learns from lots of images and texts, so it can make sense of what it sees in games, like knowing a tree is a tree because it has seen many pictures of trees with the word "tree".
- Video model: This part guesses what might happen next in the game based on what happened before. It helps SIMA understand how things in the game can change over time, like predicting a ball will fall if it's dropped.
These parts work together to help SIMA follow instructions in games it has never played before.
Training Process
SIMA learns how to play games in a few ways:
- Reinforcement learning: This is like learning from trial and error. SIMA tries different things in the game, and if something works well, it remembers to do it again. This way, it learns how to win or score points.
- Imitation learning: Here, SIMA watches how humans play and tries to copy them. This helps it learn smart ways to play without having to figure everything out by itself.
- Self-supervised pretraining: Before even starting to learn a specific game, SIMA watches lots of videos to understand common patterns, like how things generally move. This makes it easier for SIMA to learn new games faster.
By learning in these ways, SIMA gets really good at following instructions and handling different tasks in a bunch of games it hasn't seen before. It's like it can learn the rules of new games quickly, which is a big deal for artificial intelligence.
SIMA's Training Environments
SIMA learned how to be smart by playing a bunch of different video games and special computer setups made just for learning. This mix of games and setups was key to helping it figure out how to use what it knows in new situations.
Commercial Games
SIMA got to play some real video games that have big worlds and tricky parts, like:
- Goat Simulator 3
- Hydroneer
- No Man's Sky
- Satisfactory
- Teardown
- Valheim
- Wobbly Life
In these games, SIMA had to think on its feet and deal with things changing all the time. For instance, in Teardown, it learned how to carefully break things down, working with materials that act like they do in real life. Playing all these different games taught it how to see, plan, and move things around in smart ways.
Research Simulations
SIMA also practiced in special computer setups that are all about learning certain skills, like:
- Construction Lab - Putting blocks together to make stuff
- ProcTHOR - Finding its way around places that change every time
- Other simulation tasks - Getting better at interacting with things
These setups were more controlled and focused on teaching SIMA specific things, like how to move things carefully. By training in both the wide-open games and these more focused setups, SIMA learned how to be flexible and really good at certain tasks.
By playing all these games and practicing in different setups, SIMA got really good at handling new challenges. This mix of experiences helped it learn a lot about how to deal with different situations.
Features and Capabilities
SIMA has some pretty cool skills that let it understand what people tell it to do, take action, and use what it learns in one game to get better at others. Here's a closer look at what makes it a standout generalist AI agent.
Language Understanding
- SIMA is really good at figuring out what people mean when they give it instructions.
- It can handle complicated tasks and follow steps based on what someone tells it.
- This means it can do specific jobs just by listening to what you say.
Broad Action Repertoire
- It knows how to do more than 600 different things, like moving around, using objects, and navigating game menus.
- This lets it respond well in lots of different situations.
- It's flexible, so it can achieve many goals.
Multi-Environment Transfer
- SIMA can use what it learns in one game to quickly adapt to another new game.
- This helps it get better without having to learn everything all over again.
- It's smart at seeing the similarities between different games.
Continual Learning
- SIMA keeps getting smarter as it plays more games.
- It constantly learns new things, making it better at tackling complex tasks.
- Over time, it can handle longer and more difficult challenges.
Generalization Capacity
- Instead of just knowing how to do well in one specific game, SIMA can handle new and unknown challenges.
- This makes it really flexible and able to take on new tasks easily.
- Its design helps it stay good at adapting, no matter how much the games change.
By being able to understand language, do lots of actions, learn from different games, and adapt to new challenges, SIMA shows how advanced and capable it is. It's like having a smart helper that can figure out how to achieve goals in a bunch of different game worlds.
Generalization Ability
SIMA shows off its skill in learning how to handle different games and challenges without needing extra training. This part talks about how well SIMA can jump into new games it hasn't played before and how it stacks up against AI that's made for just one game.
Zero-shot Learning
- SIMA is really good at using what it knows from other games to figure out new ones without being taught again. This means it's smart enough to use its knowledge in new situations.
- For instance, when tested on 9 different games, SIMA, which was trained on 8, did almost as well on the 9th game (which it hadn't seen before) as an AI that was trained just for that game.
- This shows that SIMA can handle new games well, using what it's learned before, without needing more training.
Comparison to Specialized Agents
- When faced with over 1500 different tasks in games, SIMA did as well or better than AI trained just for those specific games.
- This proves that SIMA can use its broad experience to solve problems, rather than learning one thing at a time.
- For example, a SIMA agent trained in various tasks like building, moving around, and handling objects did better than agents made just for those tasks.
- These findings highlight how flexible and useful SIMA is compared to AI that's designed to do just one thing.
In short, SIMA's ability to learn on the fly and do well against AI made for specific tasks shows it's really good at picking up new skills. It's like a jack-of-all-trades in the world of artificial intelligence, ready to take on different kinds of challenges.
sbb-itb-bfaad5b
Ethical Considerations
Google DeepMind is working hard to make sure SIMA is a good and safe AI. They are careful about what SIMA learns, so it knows how to act in a way that's good for everyone.
Content Curation to Avoid Harmful Behaviors
-
The team made sure SIMA only played games and did activities that didn't involve any violence. This helps make sure SIMA doesn't pick up any bad habits.
-
SIMA was taught to solve problems, find its way around, and work well with others. This way, it learns to do things that can help people.
-
By keeping violent stuff out of SIMA's training, the team lowers the risk of it acting aggressively.
Promoting Helpful AI Through Positive Reinforcement
-
SIMA was encouraged to act safely and respectfully. This teaches it to value these behaviors.
-
It was given tasks that involve exploring, building things, and helping humans. This guides SIMA towards being supportive.
-
Teaching SIMA with positive feedback helps everyone trust it more because they understand why it does what it does.
By teaching SIMA in a way that focuses on being helpful and avoiding harm, DeepMind wants to make an AI that we can all trust to be good and helpful. They're making sure SIMA learns the right way, respecting people's rights.
Challenges and Limitations
Even though SIMA is doing great things, there are some big challenges it has to face. Let's talk about what's tough for it right now.
Handling Complex and Unpredictable Environments
- Sometimes, SIMA gets tripped up by really complicated games or things that happen out of the blue. Like, if a game has super tricky physics, SIMA might not do so well.
- It also finds it harder in games where stuff changes a lot without warning, such as maps that look different every time you play.
- Dealing with really complex situations or surprises while trying to make decisions quickly is still a bit of a struggle.
Data and Compute Constraints
-
To get SIMA ready and smart, it takes a ton of data and computer power, which can be pretty costly and take a lot of time.
-
SIMA is a bit of a data hog. It needed to 'play games' for more than 1,000 years during its training. Finding ways to teach it with less data could make things faster and cheaper.
-
There's also the issue of having enough computer muscle to handle SIMA's big brain in real-time.
Narrow Focus on Game Environments
- Right now, SIMA is learning a lot from video games, but real life is way more complicated. We still need to see if what SIMA learns in games can help it do things in the real world.
- Games might also teach SIMA some biases, which is something else we have to watch out for.
- The big question is whether SIMA can take what it knows from games and use it in bigger, real-world situations.
While SIMA is breaking new ground, it also shows us where we need to work harder to make AI like this useful for real-life stuff. Researchers are focused on overcoming these challenges to make AI agents that can adapt to all sorts of situations.
Future Work
SIMA is showing us it can do a lot as a generalist AI agent, but there's still more we can make it do. Here's what the researchers are looking into next:
Applications Beyond Games
- Seeing if SIMA can help with everyday tasks like housework, driving, or even in healthcare.
- Checking if what SIMA learns from games can be used in the real, less predictable world.
- Creating tests to see how well SIMA can work with physical robots.
Continual Learning
- Making SIMA smarter by teaching it more things over time.
- Helping SIMA understand and follow more complex instructions.
- Allowing SIMA to ask questions if it doesn't get something.
Sample Efficiency
- Trying to teach SIMA with less data.
- Testing different ways to make SIMA learn faster.
- Using simulations and making up data to help with training.
Interpretability
- Working on ways to show how SIMA thinks and makes decisions.
- Making it easier to see and fix any problems.
- Building trust by making SIMA's actions easier to understand.
Multi-Modal Understanding
- Expanding beyond just seeing and reading to understanding spoken commands.
- Adding more ways for SIMA to sense and interact with the world.
By improving SIMA in these areas, the goal is to create AI helpers that can be really useful for people in all kinds of situations. There's a lot more experimenting and developing to do, but SIMA is a big step towards smarter and more reliable AI.
Conclusion
SIMA is a big step forward in making smarter AI agents. It's been trained in a lot of different games and special setups, showing it can switch tasks easily without needing to learn from scratch every time.
Here are the main points to remember:
- Language understanding: SIMA is good at following detailed instructions, which means it could be used for many real-world tasks.
- Transfer learning: SIMA can use what it's learned from one game to do well in another, even better than AIs built for one specific thing. This ability to apply its knowledge to new situations is really important.
- Ethical considerations: DeepMind is making sure SIMA learns to be helpful and respectful by steering it away from violent content and encouraging positive actions. It's important to keep an eye on this.
- Challenges: SIMA still has a hard time with very unpredictable games, needs a lot of data and computing power, and is mainly learning from games. These are areas that need work.
Looking ahead, the focus is on making SIMA learn continuously, use less data, be easier to understand, understand more types of inputs, and see if it can help with tasks outside of games.
As these AI models get better, creating agents that can understand and follow instructions safely could change how we work with machines. SIMA is at the forefront of research into making AI that can be really helpful to us.
Related Questions
What is DeepMind AI used for?
Google uses DeepMind AI to make the cooling systems in its data centers work better and use less energy. This AI helps balance the cost of equipment wear and tear against the cost of cooling. DeepMind also helps Google Play suggest apps you might like, working with other AI experts at Alphabet.
Is DeepMind owned by Google?
Yes, Google bought DeepMind in 2014. DeepMind was started in London in 2010 by Demis Hassabis, Shane Legg, and Mustafa Suleyman.
How do I access Google AI?
To use AI summaries in Google Search:
- Open Chrome and log into your Google Account
- Make sure you're not using Incognito mode
- Search for something on Google
- If available, AI summaries appear at the top of the search results
To use the chat feature:
- Click "See more" under the summary
- Enter a follow-up question in the box
Who is the leader of Google AI?
John Giannandrea has been in charge of Google's Artificial Intelligence division since April 2018. He joined Google back in 1999.